Purpose: There is an emerging interest of applying magnetic resonance imaging (MRI) to radiotherapy (RT) due to its superior soft tissue contrast for accurate target delineation as well as functional information for evaluating treatment response. MRI-based RT planning has great potential to enable dose escalation to tumors while reducing toxicities to surrounding normal tissues in RT treatments of nasopharyngeal carcinoma (NPC). Our study aims to generate synthetic CT from T2-weighted MRI using a deep learning algorithm. Methods: Thirty-three NPC patients were retrospectively selected for this study with local IRB's approval. All patients underwent clinical CT simulation and 1.5T MRI within the same week in our hospital. Prior to CT/MRI image registration, we had to normalize two different modalities to a similar intensity scale using the histogram matching method. Then CT and T2 weighted MRI were rigidly and deformably registered using intensity-based registration toolbox elastix (version 4.9). A U-net deep learning algorithm with 23 convolutional layers was developed to generate synthetic CT (sCT) using 23 NPC patients' images as the training set. The rest 10 NPC patients were used as the test set (1/3 of all datasets). Mean absolute error (MAE) and mean error (ME) were calculated to evaluate HU differences between true CT and sCT in bone, soft tissue and overall region. Results: The proposed U-net algorithm was able to create sCT based on T2-weighted MRI in NPC patients, which took 7 s per patient on average. Compared to true CT, MAE of sCT in all tested patients was 97 +/- 13 Hounsfield Unit (HU) in soft tissue, 131 +/- 24 HU in overall region, and 357 +/- 44 HU in bone, respectively. ME was -48 +/- 10 HU in soft tissue, -6 +/- 13 HU in overall region, and 247 +/- 44 HU in bone, respectively. The majority soft tissue and bone region was reconstructed accurately except the interface between soft tissue and bone and some delicate structures in nasal cavity, where the inaccuracy was induced by imperfect deformable registration. One patient example was shown with almost no difference in dose distribution using true CT vs. sCT in the PTV regions in the sinus area with fine bone structures. Conclusion: Our study indicates that it is feasible to generate high quality sCT images based on T2-weighted MRI using the deep learning algorithm in patients with nasopharyngeal carcinoma, which may have great clinical potential for MRI-only treatment planning in the future.
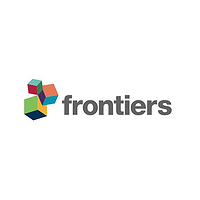
Synthetic CT Generation Based on T2 Weighted MRI of Nasopharyngeal Carcinoma (NPC) Using a Deep Convolutional Neural Network (DCNN)
Review badges
0 pre-pub reviews
0 post-pub reviews