The explosive growth of large-scale biological data enables network-based drug repositioning to be an important way of drug discovery, which can reduce the time and cost of drug discovery efficiently. Many existing approaches always construct drug-disease association network only based on some similarity measuring data for drug or disease, which ignore the impacts of different similarity measuring on predicting performance. In this study, we develop a new computational approach named BiRWDDA, which fused multiple similarity measures and bi-random walk to discover potential associations between drugs and diseases. First, multiple drug-drug similarity and disease-disease similarity are measured. Next, the information entropy of similarities measured based on different data are calculated to select proper similarities of drugs and diseases. Subsequently, improved drug-drug similarity and disease-disease similarity can be obtained by fusing similarities selected. Then, a logistic function is adopted to adjust the improved drug similarity and disease similarity. What is more, a heterogeneous network can be conducted by connecting the drug similarity network and the disease similarity network through known drug-disease associations. Finally, a bi-random walk algorithm is implemented on the heterogeneous network to predict potential drug-disease associations. Experimental results demonstrate that BiRWDDA outperforms the other state-of-the-art methods with average AUC of 0.930. Case studies for five selected drugs further verify the favorable prediction performance.
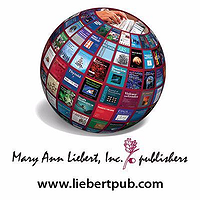
BiRWDDA: A Novel Drug Repositioning Method Based on Multisimilarity Fusion
Review badges
0 pre-pub reviews
0 post-pub reviews