In this paper we investigate the novel method, penalized joint maximum likelihood estimation (PJMLE), for estimating the parameters of the Rasch model (Rasch, 1960). Here we use joint maximum likelihood estimation (JMLE) along with elastic net penalization using the glmnet package (Friedman, Hastie, and Tibshirani, 2010) in R to obtain estimates for item difficulties and examinee abilities. Through simulation we compared the accuracy of PJMLE to conditional maximum likelihood estimation (CMLE), marginal maximum likelihood estimation (MMLE), and marginal Bayes modal estimation (MBME). We show that PJMLE successfully estimates parameters of a Rasch model when the number of items is greater than the number of examinees, which is a shortcoming of traditional estimation techniques. In addition, we further show that PJMLE performs similarly to traditional techniques when the number of examinees is greater than the number of assessment items without specifying a mixing distribution or a prior distribution.
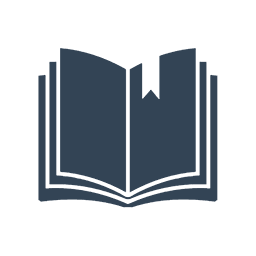
Rasch Model Parameter Estimation via the Elastic Net
Review badges
0 pre-pub reviews
0 post-pub reviews