Studies investigating individual differences in reading ability often involve data sets containing a large number of collinear predictors and a small number of observations. In this paper, we discuss the method of Random Forests and demonstrate its suitability for addressing the statistical concerns raised by such datasets. The method is contrasted with other methods of estimating relative variable importance, especially Dominance Analysis and Multimodel Inference. All methods were applied to a dataset that gauged eye-movements during reading and offline comprehension in the context of multiple ability measures with high collinearity due to their shared verbal core. We demonstrate that the Random Forests method surpasses other methods in its ability to handle model overfitting, and accounts for a comparable or larger amount of variance in reading measures relative to other methods.
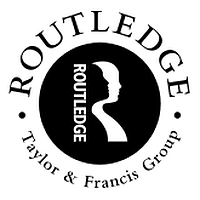
The Random Forests statistical technique: An examination of its value for the study of reading
Review badges
0 pre-pub reviews
0 post-pub reviews