In this study, we propose a novel scheme for human action recognition that combines the advantages of both spatial and temporal representations. We use shape context (SC) as pose representation in the spatial domain, and explore the temporal feature by taking into account the correlation between sequential poses within an action. In terms of the pose matching with high-dimensional data, we provide a fast matching algorithm using pyramid match kernel (PMK) based on adaptive partitioning. Additionally, this work introduces a size-pruning based longest common sub-sequence (LCSS) alignment algorithm for action sequence matching, and obtains the final cost via the decision-level fusion. Experimental results prove the viability and superiority of the fusion of two descriptors and the proposed method outperforms the majority of state-of-the-art methods on Weizmann and KTH datasets.
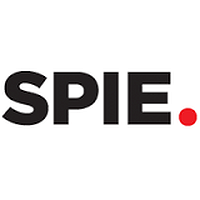
Human Action Recognition Using Spatial and Temporal Sequences Alignment
Review badges
1 pre-pub reviews
0 post-pub reviews