Brain tumor is a severe cancer and a life-threatening disease. Thus, early detection is crucial in the process of treatment. Recent progress in the field of deep learning has contributed enormously to the health industry medical diagnosis. Convolutional neural networks (CNNs) have been intensively used as a deep learning approach to detect brain tumors using MRI images. Due to the limited dataset, deep learning algorithms and CNNs should be improved to be more efficient. Thus, one of the most known techniques used to improve model performance is Data Augmentation. This paper presents a detailed review of various CNN architectures and highlights the characteristics of particular models such as ResNet, AlexNet, and VGG. After that, we provide an efficient method for detecting brain tumors using magnetic resonance imaging (MRI) datasets based on CNN and data augmentation. Evaluation metrics values of the proposed solution prove that it succeeded in being a contribution to previous studies in terms of both deep architectural design and high detection success.
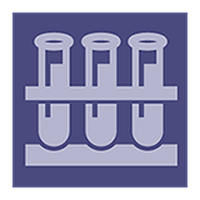
A Novel Data Augmentation-Based Brain Tumor Detection Using Convolutional Neural Network
Review badges
0 pre-pub reviews
0 post-pub reviews