In this work we propose a novel method to address illumination invariance for facial expression recognition. We propose a Deep Convolutional Network (CNN) pre-trained as a Deep Stacked Convolutional Autoencoder (SCAE) in a greedy layer-wise unsupervised fashion. The SCAE model learns to encode facial expression images and produce a feature vector with relatively similar illumination, regardless of the luminance level of the input image. Moreover, we propose fine-tuning the stacked shallow autoencoders after each one of these is trained greedily, rather than just at the end, and show that this approach significantly improves the set of illumination invariant features learnt by the SCAE. Finally, we propose the use of a variant rectifier linear unit transfer function that helps the SCAE model reduce or increase the illumination of images with high or low luminance, and show that the lower and upper bounds greatly influence classification performance. The method proposed provides an increase in classification accuracy of 4% on the KDEF dataset and 8% on the CK+ dataset.
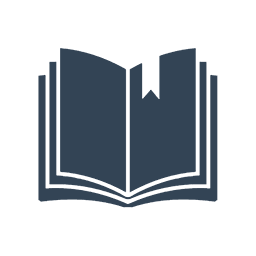
Deep Learning for Illumination Invariant Facial Expression Recognition
Review badges
0 pre-pub reviews
0 post-pub reviews